Practitioners Course in Descriptive,Predictive and Prescriptive Analytics
Created By
Deepu Philip and Amandeep Singh via Swayam
- 0
- 8 weeks long
- Swayam
- English
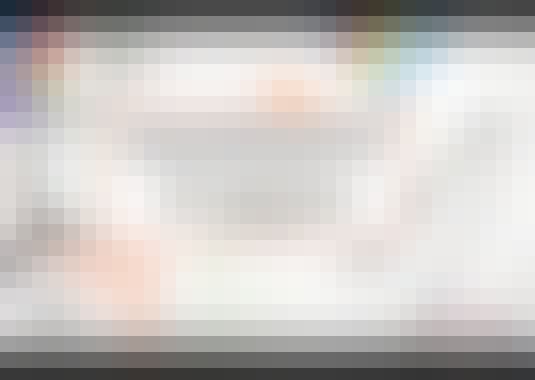
Course Overview
Data analytics is a demanding field and industry is looking for potential employees who are having a practitioners approach to data analytics. This course is aimed at providing exposure to various tools and techniques along with relevant exposure to appropriate problems so that the know-how and do-how aspect of analytics, which is required by industry can be fulfilled. The course also aims at introducing various applications with the involvement of real-life practitioners so that appropriate exposure to audience who intend to build a career in this area is possible.INTENDED AUDIENCE : Students of all Engineering and Science disciplines.PREREQUISITES : The student should have completed fivesemesters of UG Engineering or Science program.INDUSTRY SUPPORT : Analytics companies – Mu Sigma, Cisco, EXL analytics, KPMG, Ernst & Young, etc. Financial companies - CapitalOne, SBI Cap, ICICI, Amex, etc. Banking sector – SBI, UBI, Reserve Bank, HDFC, HSBC, Canara Bank, Yes Bank, etc.
Course Circullum
- Introduction to analytics
- Differentiating descriptive, predictive, and prescriptive analytics, data mining vs data analytics
- Industrial problem solving process
- Decision needs and analytics, stakeholders and analytics, SWOT analysis
- Model and modeling process, modeling pitfalls, good modelers, decision models and business expectations,
- Different types of models – overview of context diagrams, mathematical models, network models, control systems models, workflow models, capability models
- Data and its types, phases of data analysis, hypothesis and data
- Scales, relations, similarity and dissimilarity measures, sampling process, types of sampling, sampling strategies, error mitigation
- Visualization of numeric data, visualization of non-numeric data, tools available for visualizations
- Hypothesis testing, pairwise comparisons, t-test, ANOVA, Wilcoxon signed-rank test, Kruskal-Wallis test, A/B testing
- Data infrastructure, analytics and BI, data sources, data warehouse, data stewardship, meta data management
- Data and forecasting, super-forecasting, S-curve (lifecycle), moving average, exponential smoothing, error in forecasting
- Linear correlation, correlation and causality, spearman’s rank correlation, Linear regression, logistic regression, robust regression
- Hierarchical clustering (Euclidean & Manhattan), k-means clustering,Nearest neighbor, decision trees
- Basics, customer lifetime value, customer probability model,Net promoter score, survival analysis
- Product lifecycle analysis, Ansoff’s matrix, competitive map, Fundamentals of simulation, simulation types, Monte-Carlo simulation
Item Reviews - 3
Submit Reviews
This Course Include:
- Introduction to analytics
- Differentiating descriptive, predictive, and prescriptive analytics, data mining vs data analytics
- Industrial problem solving process
- Decision needs and analytics, stakeholders and analytics, SWOT analysis
- Model and modeling process, modeling pitfalls, good modelers, decision models and business expectations,
- Different types of models – overview of context diagrams, mathematical models, network models, control systems models, workflow models, capability models
- Data and its types, phases of data analysis, hypothesis and data
- Scales, relations, similarity and dissimilarity measures, sampling process, types of sampling, sampling strategies, error mitigation
- Visualization of numeric data, visualization of non-numeric data, tools available for visualizations
- Hypothesis testing, pairwise comparisons, t-test, ANOVA, Wilcoxon signed-rank test, Kruskal-Wallis test, A/B testing
- Data infrastructure, analytics and BI, data sources, data warehouse, data stewardship, meta data management
- Data and forecasting, super-forecasting, S-curve (lifecycle), moving average, exponential smoothing, error in forecasting
- Linear correlation, correlation and causality, spearman’s rank correlation, Linear regression, logistic regression, robust regression
- Hierarchical clustering (Euclidean & Manhattan), k-means clustering,Nearest neighbor, decision trees
- Basics, customer lifetime value, customer probability model,Net promoter score, survival analysis
- Product lifecycle analysis, Ansoff’s matrix, competitive map, Fundamentals of simulation, simulation types, Monte-Carlo simulation
- Provider:Swayam
- Certificate:Paid Certificate Available
- Language:English
- Duration:8 weeks long
- Language CC: